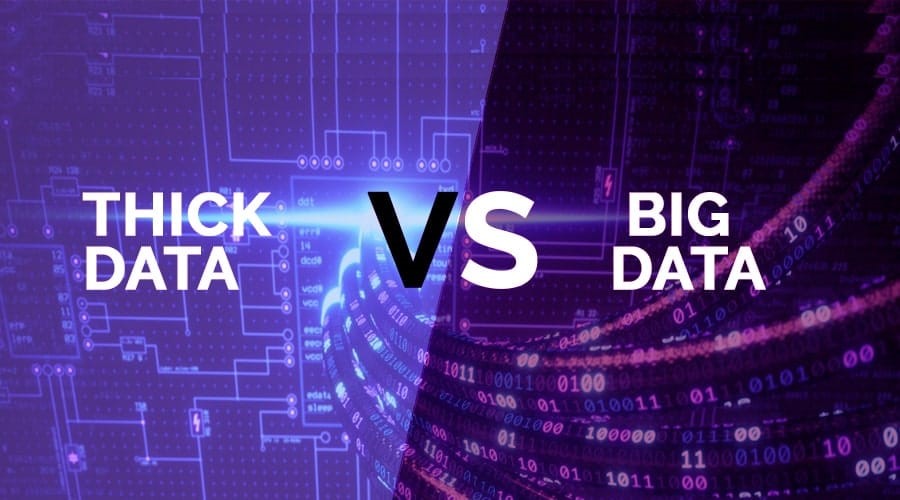
Small data can trigger well-skilled data analysts to identify them, scale them to become indicative, and put forth a prediction scheme. |
For too long, companies have relied on the assumption that large data are more reliable than small samples. That algorithms have all the answers. That quantity is the new gold. Today, this has proven to be utterly wrong.
Big Data advocacy has its downsides: focusing on how quantity affects the visualization of the analysis leads to the inevitable devaluation of the qualitative data. Whether we are using the data we now have access to on Big Data platforms or for training an AI engine, it will always lack the potential of the so called “Thick Data” – unless they are both integrated. But before we dig deep into what Thick Data can teach us, it is crucial to remind ourselves of an unquestionable truth: machines work perfectly in the application of a well-defined process, no matter how complex it can get. Even if our human brain cannot grasp it, the machine will. This is valid for platforms such as airline booking engines, core banking systems, or an atomic reactor plant management system. These operations are controlled to the minutest element, and all possibilities are calculated and optimized regularly to meet the changes. In some specific use cases – including operations like mapping public opinion on a certain topic – they are called “fault resilience systems” or “non-stop systems.” However, there are other types of wired works that are highly affected by absurdity, unpredictability, paradox and unforeseen detours. We see them all the time. |
Game changers. |
Humans are highly disruptive, extremely vocal and visible to all types of social and business networks in our highly connected planet. And as “people of this planet,” we are largely affecting the curve against algorithms.
We have tried to study the journey of these “unpredictable” phenomena in order to know what made them possible, how the ticking usually results in a sudden avalanche in such a short period of time. Alas, very little data is available to be predicted by a machine. Yet, if we look closer, this miniscule amount of information has the meaningfulness of a Greek oracle. Small data gets ridiculously trivial to be seen. However, there is a class of data that can trigger well-skilled data analysts to identify them, scale them to become iterable, and put forth a prediction scheme. There are some great examples of brands that failed and succeeded to identify and leverage thick data. If Nokia, for example, completely failed to recognize the upcoming of the smart mobile, despite their vast access to millions of data point, Netflix, on the other hand, was able to predict the emergence of a new habit based on the model of soap operas. Similarly, Samsung bet on thick data when they redesigned their TV to fulfil consumers’ expectations of having the device as a part of their household furniture and not merely of a piece of electronics. An integrative approach will allow Thick Data to uncover certain meanings in Big Data text or graphs, especially when it comes to analytics derived from text related to human experience, etymology, making Bayesian inference – a statistical method to update the probability for a hypothesis as more information emerges –a required skill to do the job. But it is anthropologists, social scientists and linguistics experts who have the right solution for they are probably more effective than any other data analysts at unearthing hidden thick data and bringing them to the surface. To this end, 21st century literacy skills such as reading, writing, and arguing make room for even more important skills: critical thinking, scientific reasoning, and multicultural awareness for they are key to solve the thick data riddle Whether it is an emotional reaction, the social context or ethnic background of an individual or a group of individuals, filling the gap between Big and Thick Data requires a depth that is not simply found in numbers. The saying “Google knows you Better than yourself” stops at the point of metaphysical prediction. Somehow, teachers can recognize a talented child in their classroom early on. Angel investors can recognize a successful idea when they see one. Marketeers looking beyond Big Data are able to expand a business in new horizons. Artificial intelligence has paved the way for us to do even better by combining these data sets to every time we seek to build a model. In fact, it will be no brain not to do. If we can imagine that an AI model that was trained using a combination of 1st party data and social media public data, the outcome will capable to predict what will happen in the physical reality with a great accuracy. And just by using social media public data |
Company
MediaSci Owner Of |
Author
Eng. Mostafa Abou-Gamrah CEO- MediaSci |